CURRENT AND PAST RESEARCH
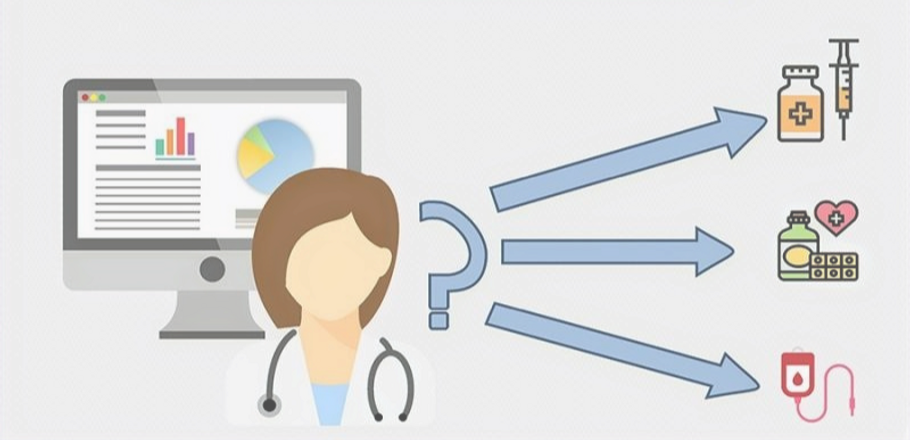
FLEXIBLE AND EASILY IMPLEMENTABLE GUIDELINES
Despite the many models developed to improve sequential decision making in medicine, those models typically cannot be implemented in their current form. To reduce the breach between theory and practice, I design sequential decision-making models that provide physicians with flexible protocols (i.e., multiple suggestions instead of rules) and with easily implementable guidelines, while continuing to improve patients’ outcomes.
​
Most mathematical models for sequentially deciding treatment medications provide a single optimal treatment choice at each decision period. Yet clinicians and their patients may prefer other alternatives that have similar effects. To benefit from physicians’ opinions and allow for patients’ preferences in the implementation of models for sequential decision problems, I proposed a new framework for identifying sets of near-optimal treatment choices.
Marrero, W. J., Lavieri, M. S., Tewari, A., Sussman, J. B., & Hayward, R. A. Data-Driven Ranges of Near-Optimal Actions for Finite Markov Decision Processes. Under review. [Link]
​
In some situations, optimal policies obtained with mathematical models may lack a structure that is intuitive to human decision makers. When interpretability is valued by practitioners, suboptimal yet interpretable policies may be preferred over uninterpretable optimal policies. To provide decision makers with interpretable strategies, my coauthors and I design and analyze a class of policies that leverage the natural interpretability of monotonicity.
Garcia, G. G., Steimle, L. N., Marrero, W. J., & Sussman, J. B. An Analysis of Structured Optimal Policies for Markov Decision Processes: The Trade-off Between Optimality and Interpretability. Under review. [Link]
​
While flexibility and interpretability are useful in clinical practice, the implementation of models may require specialized software that may not be readily available to physicians. To address this challenge, I approximated optimal treatment plans with a supervised learning model, using a large sample representative of an adult population in the US. By translating this model into a user-friendly app, I provided clinicians with fast decision support while harnessing the improved capabilities of a stochastic optimization model.
Schell, G. J., Marrero, W. J., Lavieri, M. S., Sussman, J. B., & Hayward, R. A. (2016). Data-Driven Markov Decision Process Approximations for Personalized Hypertension Treatment Planning. MDM Policy & Practice. [Link]

PERSONALIZED TREATMENT AND TESTING
FOR CARDIOVASCULAR DISEASES
Cardiovascular diseases are considered the leading cause of death in the US and worldwide. The management of cardiovascular diseases can be improved by incorporating novel procedures, such as genetic testing. However, genetic testing may be costly, and not every patient may benefit from the new information. Guidelines to inform when to perform genetic testing and how genetic information may influence patients’ treatment are valuable tools across medical domains.
To understand the clinical and policy implications of genetic testing in cardiovascular diseases, I developed a thoroughly validated simulation model to evaluate the impact of genetic information across different populations in the US.
Marrero, W. J., Lavieri, M. S., & Sussman, J. B. A Simulation Model to Evaluate the Implications of Genetic Testing in Cholesterol Treatment Plans. Proceedings of the 2019 Winter Simulation Conference, N. Mustafee, K.-H.G. Bae, S. Lazarova-Molnar, M. Rabe, C. Szabo, P. Haas, and Y.-J. Son, eds. [Link].
​
Building upon this work, I designed a framework that combines dynamic programming with value of information analysis to simultaneously determine optimal treatment and genetic testing decisions.
Marrero, W. J., Lavieri, M. S., & Sussman, J. B. (2021) Optimal Cholesterol Treatment Plans and Genetic Testing Strategies for Cardiovascular Diseases. Health Care Management Science. [Link].
​
This work serves as an aid for clinicians and health insurance policies by identifying patients who will likely benefit from genetic information.

SUPPLY, DEMAND, AND ALLOCATION OF ORGANS FOR TRANSPLANTATION
Organ transplantation is a life-saving intervention for patients with organ failure. Unfortunately, the demand for organs greatly outweighs its supply. Population shifts play a vital role in the supply, demand, and allocation of organs for transplantation.
​
Using data from the Organ Procurement and Transplantation Network, I created a population-based heuristic and a stochastic simulation model to predict liver supply and demand.
Parikh, N., Hutton, D., Marrero, W.J., Sanghani, K., Xu, Y., & Lavieri, M. S. (2015). Projections in Donor Organs Available for Liver Transplantation in the United States: 2014-2025. Liver Transplantation. [Link]
Parikh, N. D., Marrero, W. J., Wang, J., Steuer, J., Tapper, E. B., Konerman, M., Singal, A. G., Hutton, D. W., Byon, E., & Lavieri, M. S. (2017). Projected Increase in Obesity and Non-Alcoholic Steatohepatitis-Related Liver Transplantation Waitlist Additions in the United States. Hepatology. [Link]
​
To evaluate the impact of allocation policies on liver supply and demand, I developed a supervised learning model that characterizes variables that contribute to geographic inequity.
Parikh, N. D., Marrero, W. J., Sonnenday, C. J., Lok, A. S., Hutton, D. W., & Lavieri, M. S. (2017). Population-Based Analysis and Projections of Liver Supply Under Redistricting. Transplantation. [Link]
​
Together, these models provide transplantation policymakers with insights into the potential future of the US organ donation system.
Besides modeling the supply, demand, and allocation of livers, I designed machine learning frameworks to predict the likelihood of kidney discard before transplantation and how many organs one can expect to recover from a deceased donor.
Marrero, W. J., Naik, A. S., Friedewald, J. J., Xu, Y., Hutton, D. W., Lavieri, M. S., & Parikh, N. D. (2016). Predictors of Deceased Donor Kidney Discard in the United States. Transplantation. [Link]
Marrero, W. J., Lavieri, M. S., Guikema, S. D., Hutton, D. W., & Parikh, N. D. (2021). A Machine Learning Approach for the Prediction of Overall Deceased Donor Organ Yield. Surgery. [Link]​
​
To understand the potential effect of changes to the organ donation metrics and practices across the US, I contributed to the evaluation of organ donation rates and the possible impact of presumed consent.
DeRoos, L. J., Zhou, Y., Marrero, W. J., Tapper, E. B., Sonnenday, C. J., Lavieri, M. S., Hutton, D. W., & Parikh, N. D. (2020). National Organ Donation Rates and Organ Procurement Organization Metrics. JAMA Surgery. [Link]
DeRoos, L. J., Marrero, W. J., Tapper, E. B., Sonnenday, C. J., Lavieri, M. S., Hutton, D. W., & Parikh, N. D. (2019). Estimated Association Between Organ Availability and Presumed Consent in Solid Organ Transplant. JAMA Network Open. [Link]
​
These modeling frameworks offer government agencies additional tools to evaluate the effectiveness of organ procurement practices.